Machine Learning Can Help Predict Length of Hospital Stay for Lupus Flares
A new study shows that machine learning (ML) can be used to help predict length of hospitalization for people admitted for lupus flares. Length of stay (LOS) is an important measure for people with systemic lupus erythematosus (SLE), as it is directly associated with disease severity and cost of care.
In this study, researchers looked at a sample of 5,831 people hospitalized for lupus flares from the National Inpatient Sample Database. Four ML techniques were used to predict the amount of time a patient was hospitalized and assess which features had the most impact on that amount of time. Researchers found:
- Central line placement, spinal tap, acute dialysis, and kidney biopsy have the most impact on LOS – these were positively associated with hospital stays over 14 days
- Other significant features include renal involvement and infectious comorbidities such as sepsis
- Demographic characteristics such as age, race and income are in the top 20 positive predictors for LOS – of note, non-white races and lower income were both significantly associated with LOS
- Long-term steroid and biologic use before admission to the hospital are more likely to predict a shorter stay (less than 14 days)
This study helps researchers further understand the relationship between LOS and SLE outcomes, which is critical for improving patient care and bringing down healthcare costs. Future studies are needed to further explore the impact of interventions aimed at reducing disparities in LOS and improving outcomes for all, regardless of demographics, to promote health equity. Learn more about lupus research.
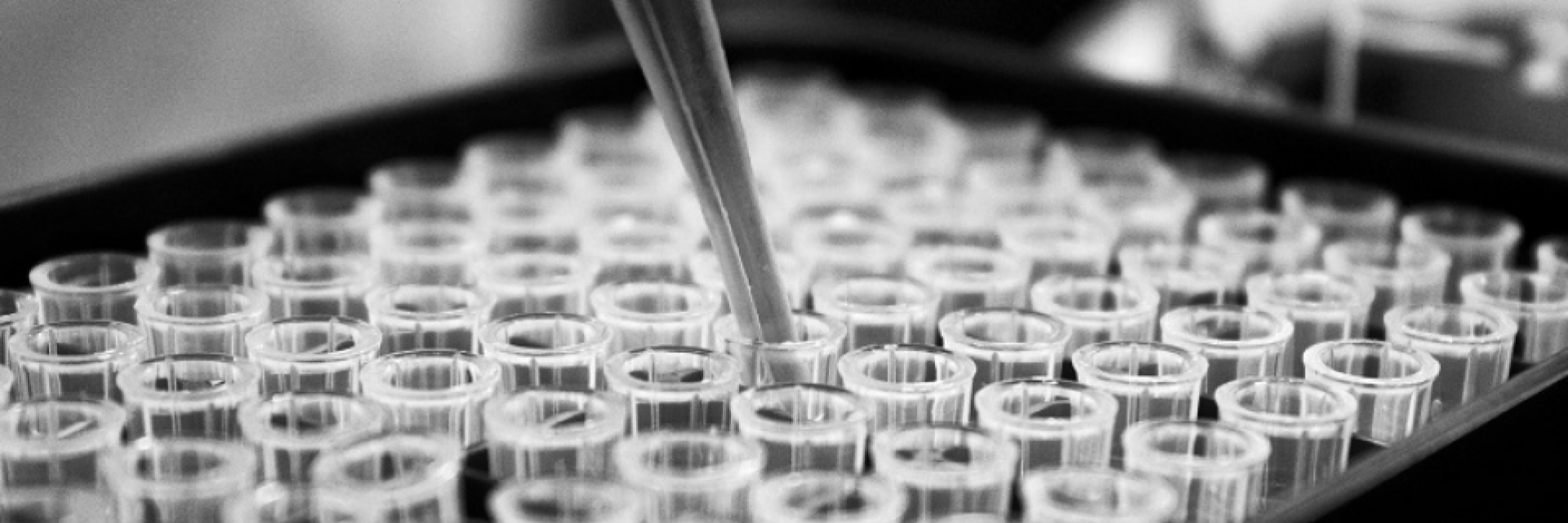
Interested in getting research like this straight to your inbox? Subscribe to our bimonthly Inside Lupus Research email for all the latest.